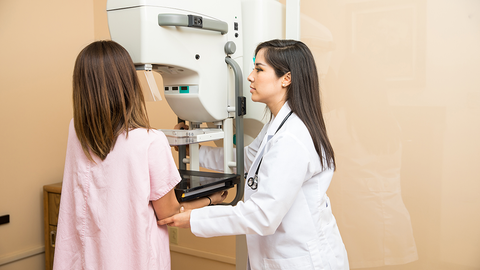
A deep learning algorithm that pulls biomarkers from screening mammograms can outperform traditional risk assessment models when predicting a patient’s individual breast cancer risk.
A team from Massachusetts General Hospital, led by breast radiologist Leslie Lamb, M.D., M.Sc, will present their findings during the Radiological Society of North America (RSNA) annual meeting this week.
“Traditional risk assessment models do not leverage the level of detail that is contained within a mammogram,” Lamb said. “Even the best existing traditional risk models may separate sub-groups of patients but are not as precise on the individual level.”
In fact, she said, current traditional risk assessment models rely on a sliver of patient data, including family history, prior breast biopsies, and hormonal and reproductive history. Breast density is the only bit of information pulled from screening mammograms that is incorporated into these models.
Related Content: Expanded Risk Model Identifies Women Who Need Additional Breast Cancer Screening
Making use of only that one piece of information undermines the potential ability to give women individualized assessments of their personal risk, the team asserted.
“Why should we limit ourselves to only breast density when there is such rich digital data embedded in every woman’s mammogram,” said Constance D. Lehman, M.D., Ph.D., senior study author and division chief of breast imaging at MGH. “Every woman’s mammogram is unique to her just like her thumbprint. It contains imaging biomarkers that are highly predictive of future cancer risk, but until we had the tools of deep learning, we were not able to extract this information to improve patient care.”
To develop this deep learning algorithm, which has been externally validated in Sweden and Taiwan, Lamb’s team gathered data from women with a personal history of breast cancer, implants, or prior biopsies who were seen at one of five MGH breast screening locations. Overall, they included 245,753 consecutive 2D digital bilateral screening mammograms performed in 81,818 patients between 2009 and 2016. Of these exams, 210,819 studies from 56,831 patients were used for training, 25,644 from 7,021 women were used for testing, and 9,290 exams from 3,961 patients were used for validation.
Related Content: AI Risk Score Predicts Breast Cancer Risk Better Than Mammographic Density
The team, then, used statistical analysis to figure out how well the deep learning model performed in predicting future breast cancer within five years of the index mammogram when compared to an existing commercially available risk assessment model, particularly the Tyrer-Cuzick version 8. The outperformance was significant, the team said – the deep learning model had a predictive rate of 0.71, but the traditional model only reached a 0.61 rate.
For additional RSNA coverage, click here.
“Our deep learning model is able to translate the full diversity of subtle imaging biomarkers in the mammogram that can predict a woman’s future risk for breast cancer,” Lamb said, noting that future studies are planned with larger African American and minority populations.
Ultimately, she said, using a deep learning model can save both time and money.
“Traditional risk models can be time-consuming to acquire and rely on inconsistent or missing data,” she said. “A deep learning image-only risk model can provide increased access to more accurate, less costly risk assessment and help deliver on the promise of precision medicine.”
For more coverage based on industry expert insights and research, subscribe to the Diagnostic Imaging e-Newsletter here.
"traditional" - Google News
November 30, 2020 at 07:04AM
https://ift.tt/2VfIzP3
Deep Learning Model Outperforms Traditional Breast Cancer Risk Assessment - Diagnostic Imaging
"traditional" - Google News
https://ift.tt/36u1SIt
Shoes Man Tutorial
Pos News Update
Meme Update
Korean Entertainment News
Japan News Update
Bagikan Berita Ini
0 Response to "Deep Learning Model Outperforms Traditional Breast Cancer Risk Assessment - Diagnostic Imaging"
Post a Comment